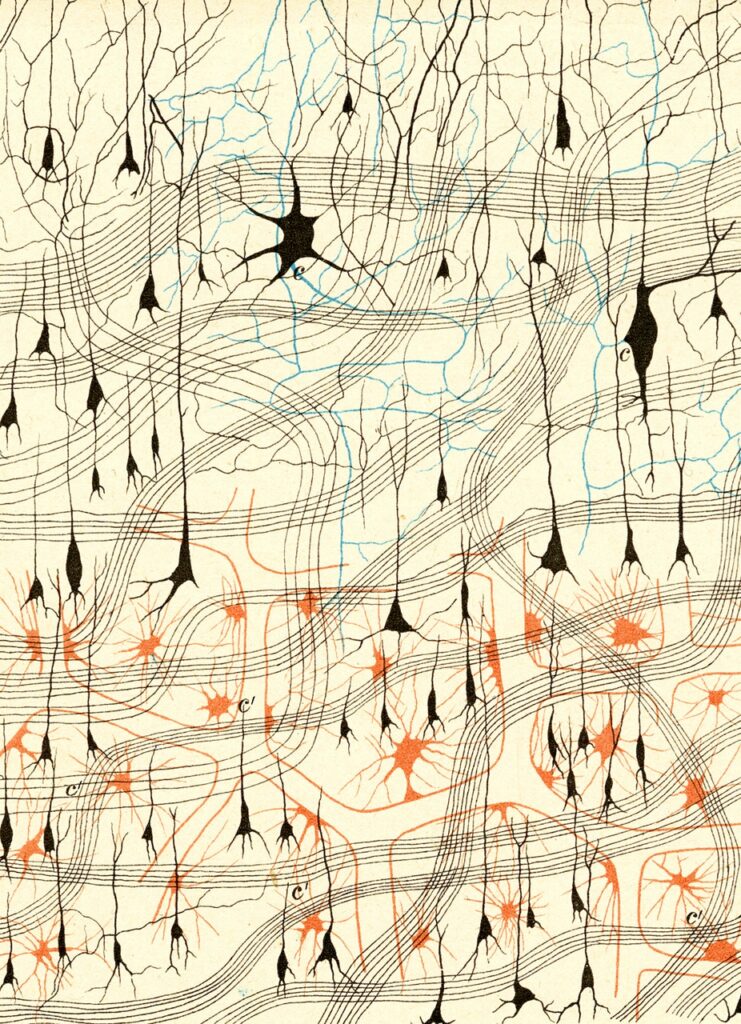
This article discusses the possible impact of AI on the environment, economy and society and reviews efforts to govern related developments. It was initially published in Special Issue Vol. 36/O1 of the journal OekologischesWirtschaften (Ecological Economy) and edited for this magazine.
Advances in AI effectiveness have made its application ubiquitous in many economic sectors. Whether speech or facial recognition, computer games or social bots, medical diagnostics or predictive maintenance or even autonomous driving, many actors expect opportunities not only for product innovations and new markets but also for new research perspectives. Economic and political actors alike expect AI-based systems and applications to contribute positively to sustainability goals (Jetzke et al. 2019). These include, for example, the opportunities offered by AI for improving the management of smart grids, and transport infrastructures, for conducting more precise earth observation, for creating new weather warning and forecasting systems, or for enhancing solutions for waste and resource management.
Do we really talk about ‘artificial intelligence’?
AI is generally used to describe machines (usually computers) that mimic cognitive functions, for example by reproducing human decision-making structures through functions with trainable parameters. AI research typically addresses problems of reasoning, knowledge representation, planning, learning, natural language processing, and perception. While the comprehensive reproduction of human intelligence, usually referred to as ‘strong AI’ (e.g. CogPrime, cf. Goertzel et al. 2014), is still far from real-world application, ‘weak AI’, such as deep learning, is now increasingly found in numerous applications. These forms of ‘weak AI’ are also described as computational intelligence (Poole et al. 1998) or intelligent agents (Russell / Norvig 2003) as they allow decision preparation, and even implementation, to be delegated to computers. Those algorithmic decision-making processes can include anything from highly complex neural networks to quite simple software applications that calculate, weigh up and sort data based on simple rules (cf. AlgorithmWatch 2018). In our text, we focus on weak AI, for example decision-making with more or less complex data-learning algorithms.
Yet even weak AI-based systems and applications (in the following we will only use the term AI) allows computers to partly take over human decision-making and to fully automate systems’ management as, for example, when supporting architects in constructing new buildings, doctors in making medical decisions, recruiters in selecting new employees or assigning Uber-drivers to trips. However, AI uses data and algorithmic reasoning to make recommendations that are not transparent – and that in many cases not even AI-researchers fully understand.
Therefore, the current rise of AI raises questions of what form of comprehensive political rules are needed to ensure the human-centred and ecological use of those technologies. This article helps to shed light on the social, ecological, and economic implications of AI and on what guidelines, rules and regulations need to be discussed and implemented to address sustainability concerns.
There are two interlinked perspectives of how to relate AI to sustainability. The first one refers to employing AI in areas that contribute to socially and ecological desirable developments, such as climate protection or education (AI for sustainable development). We investigate the second perspective, which refers to developing, implementing and using AI in a way that minimizes negative social, ecological and economic impacts of the applied algorithms (sustainable AI).
Rules for responsible AI
Over the last decade, a number of issues concerning the societal implications of AI and the respective Algorithms have been discussed intensively, mainly under the concept of ethical AI guidelines (see Jobin et al. 2019 for an overview). Aspects such as transparency, trustworthiness, autonomy and data protection are discussed – while the consideration of ecological and equitable aspects of AI is, by and large, still lacking.
The AI Ethics Global Inventory (AlgorithmWatch 2020) identifies more than 160 rules or guidelines published by diverse actors including not only NGOs, business associations and trade unions but also various governments and intergovernmental organizations such as the United Nations and the European Union (EU). The rules for using AI can range from recommendations over voluntary commitments to binding regulations, some of which are currently developed at the EU-level.
For example, the NGO iRights Lab developed the ‘Algo.rules’, a catalogue of nine rules that should be adhered to in order to enable and facilitate a socially beneficial design and appropriate use of Algorithmic Systems. These rules include aspects such as strengthening competencies of those who develop, operate and/or make decisions regarding the use of algorithmic systems or define responsibilities in a transparent and reasonable way and not transfer the responsibility to the algorithmic system itself, users or people affected by it. Other rules define that objectives and expected impact of the use of an algorithmic system must be documented and assessed prior to implementation, the application must have been tested, and the use of an algorithmic system must be identified as such (Bertelsmann Stiftung / i.Rights Lab 2020). The compliance with these rules should be ensured by design when systems are being developed.
The EU has published a White Paper providing a general regulatory regime for developing and implementing AI. The White Paper is based on recommendations from the High Level Expert Group on AI, which published its Ethical Guidelines for a Trustworthy AI in April 2019 (AI HLEG 2019). The White Paper focuses on creating ‘ecosystems for excellence’, as well as on trust and a safe and trustworthy use of AI. An ‘ecosystem for excellence’ mainly refers to the cooperative action of EU member states to maintain Europe’s leading position in research, promote innovation, expand the use of AI, and achieve the objectives of the European Green Deal. The ‘ecosystem for trust’ is based on existing law, in particular on the provisions of the General Data Protection Regulation (GDPR) and the directive on data protection in law enforcement.
The White Paper’s intended EU regulatory regime would apply extended regulation only to AI that contains a particular risk potential with regard to protection of safety and consumer and fundamental rights (European Commission 2020). The White Paper proposes defining high risk cumulatively: AI used in ‘high risk’ sectors such as health, transport, police or jurisdiction and AI application that poses significant risks, i.e. the purpose of the respective AI. Regarding for example the health sector there is a difference between using AI for appointment scheduling in hospitals or using AI for medical diagnosis. The commission states that AI for the purpose of “remote biometric identification and other intrusive surveillance technologies would always be considered ´high-risk`” (European Commission 2020: 18).
In Germany, the Data Ethics Commission advocates a five-level risk-based regulatory regime, ranging from no regulation for the lowest risk AI to a complete ban for the highest risk AI, such as autonomous weapon systems. Finally, the EU announced in the White Paper it would foster the development of AI for climate change mitigation and for the protection of natural resources. Considering AI as an enabler, the EU aims to combine the ‘European Green Deal’ with the development of ‘Trustworthy AI made in Europe’ (European Commission 2020).
Two general sustainability challenges for AI
Two questions are particularly relevant with regard to sustainable development and AI. First, are the data sets generally used to train AI algorithms at all useful for transforming existing production and consumption patterns towards sustainability? The challenge here is that existing data sets provide diverse information about the past but hardly any information about desired futures. Therefore, AI trained on historical data sets may be biased to reproduce the unsustainable status quo. To give an example: AI algorithms can optimize traffic flow management in cities or in logistics and thereby contribute to reducing fuel consumption per kilometre driven. But (how) can existing data sets train AI to help sustainably transform the transport system as a whole, e.g., to make it less car-dependent?
Existing data sets provide diverse information about the past—but hardly any information about desired futures.
Every weak AI or algorithmic system is only as good as the utility function it seeks to optimize and the data that it is based upon. That is, sustainability goals, such as reducing car traffic not only have to be implemented into the utility function of the respective algorithms but in the political regulations and conditions, as well.
Second, how can AI-supported sustainability transformations of production and consumption patterns be democratically legitimized? To stay with the transport example: Should AI-based recommendations be trimmed to inscribe preferences for ecological means of transport (bicycle, bus and train) over less ecological means of transport (car, taxi, plane)? Without a doubt, other criteria such as travel time or safety are decisive for users when choosing a mode of transport. A situation may arise in which users cannot clearly understand which criteria (i.e., which specific set of preferences) are used in an AI-based recommendation system to make or propose decisions. To avoid sustainability transformations becoming visible only through the output of the systems, the algorithms must be as transparent as possible, as should information on the algorithm training data. This inclusion could prevent the data analysis from reproducing the discriminatory and unsustainable patterns existing in society (Wolfangel 2018).
Resource and energy intensities of AI
The discussion about AI opportunities and risks has only recently begun to take into account how much energy and resources AI itself consumes for computing. The training period of an artificial neural network (ANN), devour particularly large amounts of energy. A study using BERT, an ANN used for speech recognition, determined that the training period alone resulted in 0.65 tons of CO2 being emitted (Strubell et al. 2019). This amount corresponds to the emissions generated from a return flight between Berlin and Madrid. However, the study’s frequently cited result that “training a single AI model can emit as much carbon as five cars in their lifetimes” (Hao 2019) is incorrect (cf. Lobe 2019). This often-cited amount of 313 tons of CO2 refers to neural architecture search, very different from training a ‘typical’ ANN. Notwithstanding, the training of increasingly complex deep learning models can be expected to require more compute and hence even more electricity (see graphic).
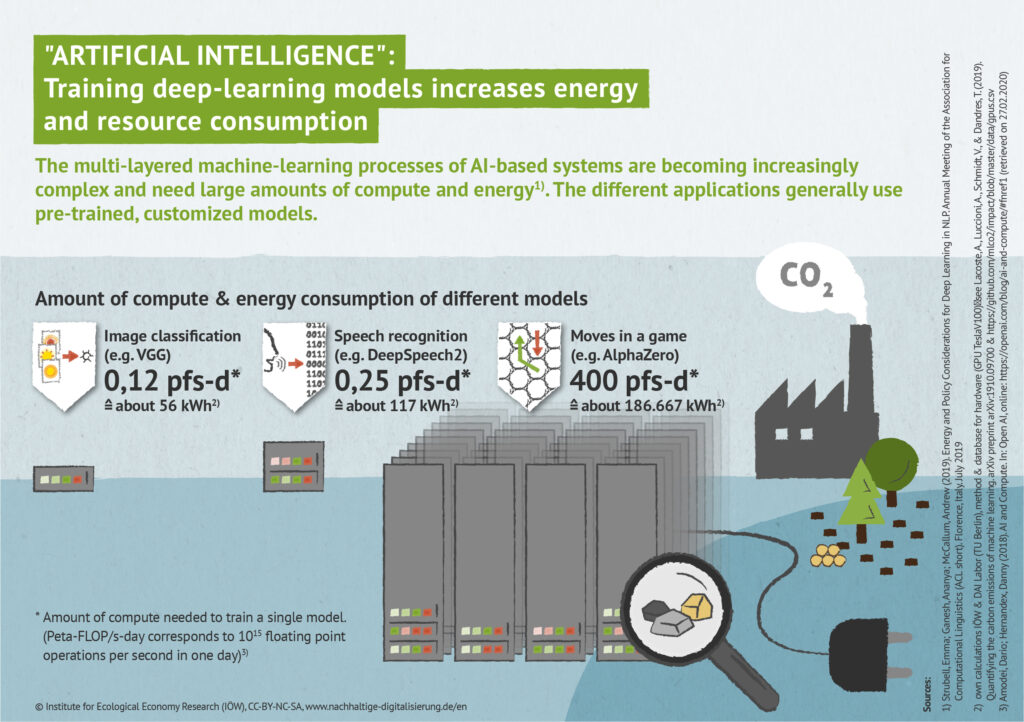
Ensuring that AI – particularly those used for sustainability purposes – generate net benefits by reducing energy and emissions requires assessing whether the energy consumed in the training and use phases justifies the intended effects. Until now, most AI has not been used solely to improve sustainability but applied in other fields ranging from optimizing online advertising to industrial production or medical technology. That is, the impact which derives from this energy intensive training process is highly dependent on the application. How much additional energy consumption of future, yet-to-be-developed, AI can societies justify when, at the same time, they have committed to the UNFCCC Paris Declaration and want to achieve the 1.5 degrees Celsius climate change goal? It appears evident that AI development must be related more strongly to socially and ecologically relevant challenges (Jetzke et al. 2019).
Moreover, the development of applications for automated decision-making, data processing, tracking, or recommendation systems should take into account alternative methods and tools to calculate, predict and classify data. For example, the accuracy of an ANN for learning a new task involves an energy-intensive trial-and-error process (Strubell et al. 2019) that sometimes only leads to a comparatively small increase in network performance. In certain applications that currently use AI, statistical analysis methods, such as linear regressions or simple neural networks, with a significantly lower energy consumption can lead to similar results. In addition to high power consumption, the AI’s material requirements pose further ecological challenges due to the hardware used in data centres and end-user devices is extremely resource-intensive.
How to enhance the ecological sustainability of AI
Concerning general sustainability criteria for software, Naumann et al. (2011) developed a comprehensive catalogue of criteria that take into account an application’s entire software life cycle – from the original coding, over its use, to deinstallation. Moreover, the software criteria cover the kind of hardware a certain software requires. These considerations were further developed and extended to modern software architectures by also taking into account the electricity load on a remote server, the local client, or the network as a transport medium (Gröger et al. 2018). Applying a whole-system approach allows for sharpening the view for indirect effects, also referred to as higher order effects of ICT (Pohl et al. 2019) which relate to behavioural and structural changes, that occur due to new business models or the transformation of everyday practices, such as online shopping.
Schwartz et al. (2019) propose criteria that are suitable for assessing the ecological effects of AI and include criteria such as CO2 emissions, power consumption, training duration, number of parameters, and number of floating-point operations (FLOP). However, these criteria raise the question of the type of measurement as different computers consume different amounts of energy for the same operation. The current project ‘Sustainability Index for Artificial Intelligence’, a cooperation between the advocacy organisation AlgorithmWatch, the Institute for Ecological Economy Research (IÖW) and Distributed Artificial Intelligence Laboratory (DAI) at TU Berlin, aims to develop a comprehensive set of sustainability criteria for AI-based systems and establish particular guidelines for sustainable AI-development.
‘Sustainability Index for Artificial Intelligence’ aims to develop a comprehensive set of sustainability criteria for AI-based systems and establish particular guidelines for sustainable AI-development.
In addition to guidelines for developing and applying AI, politics can set appropriate regulatory frameworks. Oftentimes, their focus is not specifically on AI only, but include wider technological developments that are AI-related, such as the GDPR, ePrivacy directive, energy prices, or the pricing of carbon emissions. Thus, CO2-taxes on electricity could make the development of less complex and energy-saving models more attractive – incentivizing software developers and their clients to balance energy costs with performance benefits.
One of the most relevant steps, not only for the development of AI but also for developing data-based applications in general, is the promotion of green cloud computing and green data centres, as argued by Köhn et al. (2020). Data centres should be legally bound to provide energy certificates that provide information on their energy consumption and performance. By collecting this information in a central data register, establishing and expanding new data centres can be better planned and promoted. Furthermore, cloud services should provide information on their ecological impact by way of a CO2-footprint per service unit (e.g., per hour, per year). AI-developers should be obliged to report on the CO2 emissions of the AI-models used, e.g., by way of initiatives such as the ‘CO2 Impact Calculator’. Creating greater transparency would also incentivize cloud providers to offer more climate-friendly services.
Finally, overarching incentive instruments for reduced energy and resource consumption, such as taxes on CO2 or resource, a sustainability-oriented national (or EU-wide) resource policy, or public procurement guidelines could provide further incentives to enhance the development and use of the most energy- and resource-efficient AI, and for consumers to choose alternatives to AI where possible.
Regulation of market power and monopolies
The interests of actors driving the creation of new AI applications and markets will considerably determine whether and to what extent AI actually supports a transition towards sustainable production and consumption patterns. The majority of AI today pursues the aim to personalise services, forecasting customers’ purchasing interests and optimizing online marketing and advertisements (Heumann / Jentzsch 2019). These applications intend to increase both individual and societal levels of consumption, which in many countries are already unsustainably high.
The marketing of AI generates high revenues. For example, in the market segment of multi-purpose assistants such as Siri or Alexa, revenues of USD 11.9 billion are forecast for 2021 (Hecker et al. 2017). Large tech companies leading these markets are currently using AI to enhance their market power and competitive advantages. The related dominance of a few global tech corporations, first and foremost Google, Amazon, Facebook, Apple, Microsoft and a few others, will most likely continue with the development and commercialization of AI in the future (Staab / Butollo 2018). Due to the high importance of big data for AI, tech corporations are reluctant to make ‘their’ data openly available to competitors, while using mergers and acquisitions to gain access to further data sources.
Because the control over large amounts of data functions as a central barrier to AI market entry (Wiggerthale 2019), existing competitive challenges associated with large platform monopolies are likely to be aggravated in coming years. Since all large tech corporations are shareholder-owned, and hence have to service capital interests on financial markets, it is questionable whether increasing market concentration will help AI business models that place people and the planet over profits. Today’s antitrust laws are not suited to counteracting this development. Since monopolies are legal under competition law, antitrust laws only take effect when companies abuse their market power to deprive competitors, exploit market partners, or raise unjustifiably high consumer prices.
Large concentrations of data in the hands of few actors is by no means a topic for antitrust and competition laws. If it were a topic, large tech companies would no longer be able to take over AI competitors and start-ups. Antitrust law worldwide should be reformed accordingly. For example, the initiative ‘Restrict Corporate Power’ urges the German government to prohibit dangerous monopolies in the digital economy under cartel law and to create legislation allowing them to be disbanded. To counteract the concentration of power on a few large platforms, independent data collaborations are being discussed (Heumann / Jentzsch 2019). According to research by the Foundation New Responsibility, numerous approaches already exist for jointly using data platforms or pools, but so far with little success. The state can support data cooperation by providing a distinct regulatory regime and more legal security, for example with regard to liability and data protection. Moreover, to curb data monopolies and, at the same time, make data more openly accessible for socially- and sustainability-oriented companies and other causes, governments could establish public data trusts to function as intermediaries between those actors that generate data and those that intend to use it (Staab 2019). Different data trusts could be established for energy-related, mobility-related, or (smart) city-related data.
To protect the interests and safety of consumers, necessary regulatory frameworks could also include liability issues (European Commission 2020). Due to the difficulty of tracing potentially problematic decisions made by AI, individuals harmed by an AI may not have access to evidence crucial for a court case. Relevant EU legislation should be adapted, and AI standardization should ensure that processes are comprehensible and accessible for evidence (German Bundestag 2020).
Finally, discussions are underway about a digital tax at the national or European level that would ensure value creation in the digital economy also contributes to financing public tasks. From a sustainability perspective, a further step would be discussing the allocation of funds solely for sustainable AI.
It is key to ensure that the broad application of AI-based systems, which opinion leaders expect in the future, will be implemented sustainably. Modern data-driven architectures and specialized hardware and the peculiarities of machine learning and AI still lack suitable sustainability criteria. Achieving sustainable AI needs comprehensive guidelines, rules, and regulations. These should ensure a reasonable and purposeful use of AI with regard to the desired objectives, ecologically sustainable, transparent and free from exclusion and discrimination.
References
See original post for full references.
Authors
Friederike Rohde, Maike Gossen and Josephin Wagner are researchers at the
Institut für ökologische Wirtschaftsforschung (IÖW). Tilman Santarius is Professor of Social-Ecological Transformation and Sustainable Digitalization at the Technical University of Berlin and the Einstein Center Digital Futures.